International Journal of Applied Research in Mechanical Engineering IJARME
ISSN: 2231-5950
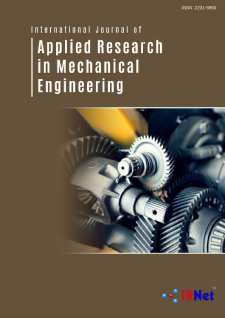
Abstracting and Indexing
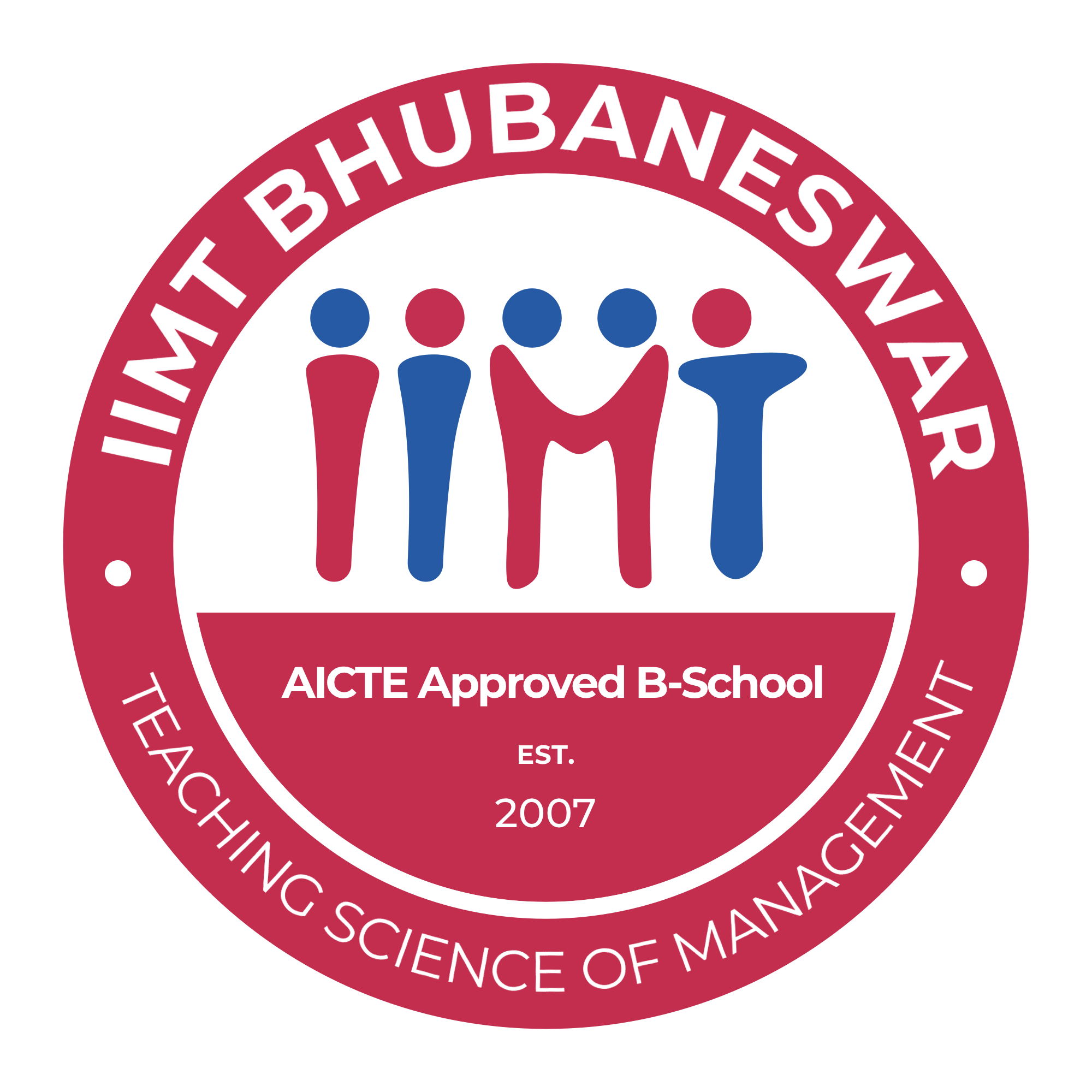
IJARME
Vibration analysis of bearing for fault detection using time domain features and neural network
Manish Yadav
Department of Electrical Engineering,Madhav Institute of Technology and Science Gwalior, India
Dr. Sulochana Wadhwani
Department of Electrical Engineering,Madhav Institute of Technology and Science Gwalior, India,
Abstract
Ball bearings are among the most important and frequently encountered components in the vast majority of rotating machines, their carrying capacity and reliability being prominent for the overall machine performance. Fault detection and diagnosis in the early stages of damage is necessary to prevent their malfunctioning and failure during operation. This paper presents fault detection of ball bearing using time domain features of vibration signals. The vibration signals are recorded at bearing housing of 5hp squirrel cage induction motor. These extracted features are used to train and test the neural network for four bearing conditions namely: Healthy, defective Outer race, defective Inner race and defective ball fault condition. The experimental observation shows that the proposed method is able to detect the faulty condition with high accuracy.
Recommended Citation
[1] Boving K. G., “NDE Handbook for Condition Monitoring”, Butterworth’s, Denmark 1989. [2] Chow M. Y., Sharpe R. N. and Hung J. C., “On the Application and Design of Artificial Neural Networks for Motor Fault Detection -Part I”, IEEE Trans. on Industrial Electronics, Vol. 40(2), pp. 181-188, April 1993. [3] H.B Demath, M. Beale, “Neural network toolbox for use with MATLAB” User’s guide, The math works Inc. Natick M.A, USA,2004 . [4] Y. Lei, Z. He, Y. Zi, Q Hu, “Fault diagnosis of rotating machinery based on a new hybrid clustering algorithm,” International J of Advanced Manufacturing Technology, vol. 35, 2008, pp. 968-977. [5] B. Sam anta, K. R. Al-balushi, “Artificial neural network based fault diagnostics of rolling element bearings using time-domain features,” Mechanical Systems and Signal Processing, vol. 17(2), 2003, pp. 317-328. [6] B. Li, G. Goddu, M. Chow, “Detection of common bearing faults using frequency-domain vibration signals and a neural network based .approach,” Proceedings of the American Control Conference, Philadelphia, Pennsylvania, June 1998, pp. 2032-2036. [7] D. M. Yang, A. F. Stronach, P. Mac Connell, “Third-order spectral techniques for the diagnosis of motor bearing condition using artificial neural networks,” Mechanical Systems and Signal Processing, vol.16(23), 2002, pp. 391- 411. [8] B. S. Yang, T. Han, J. L. An, “ART-KOHONEN neural network for fault diagnosis of rotating machinery,” Mechanical Systems and Signal Processing, vol. 18, 2004, pp. 645-657. [9] S. Abbasion, A. Rafsanjani, A. Farshidianfar, N. Irani, “Rolling element bearings multi-fault classification based on the wavelet denoising and support vector machine,” Mechanical Systems and Signal Processing, vol. 21, 2007, pp. 2933-2945. [10] C. Bishop, Neural networks for pattern recognition, Oxford University Press, New York, 1995. [11] M.R. Rafimanzelat, B.N, Arabbi, and E. Khosroshabi, “Induction machine broken bar detection using neural network based classification,” in Proc. IEEE conf. Cybern. Intell. Syst., 2004,vol. 2,pp. 1362-1367 [12] S. Nandi and H.A. Toliyat, “condition monitoring and fault diagnosis of electrical machines –A review, in Proc. IEEE conf. Ind, Appl., vol. 1, pp.197-204 [13] Alguindigue I.E., wicz-Buzak, A.E., and Uhrig R.E.”Monitoring and diagnosis of rolling element bearing wring Artificial neural network.” in Proc. IEEE Trans. on Ind, Electronics, vol. 40, 1993, pp.209-217. [14] Norton M.P and Karcuzb D.G., “ Fundamentals of noise and vibration analysis for engineers” Cambridge university press, UK,2003 [15] T. I. Laine, K. W. Bauer, “Feature selection assessment and comparison using two saliency measures in an Elman recurrent neural network, ”Proceedings of theInternational Joint Conference on Neural Networks, IEEE Press, vol. 4, July 2003, pp. 2807- 2812. [16] R. J. Alfred son and J. Mathew, "Time Domain Methods for Monitoring the Condition of Rolling Element Bearings, “presented at Mechanical Engineering Transactions, 1985. [17] N. Arthur and J. Penman, “Induction Machine Condition Monitoring with Higher Order Spectra,” IEEE Trans. on Ind. Electronics, vol. 47, no. 5, Oct. 2000, pp.1031-1041. [18] P. Vas, “Parameter Estimation, Condition Monitoring, And Diagnosis of Electrical Machines,” Clarendon Press, Oxford,1993. [19] W.R. Finley, M.M. Hodowanec and W.G. Holter, “An Analytical Approach to Solving Motor Vibration Problems,” IEEE Trans. on Ind. Appl., vol. 36, no. 5, Sep. /Oct. 2000, pp. 1467-1480. [20] F.C. Trutt, J Sottile and J.L. Kohler, “On-Line Condition Monitoring of Induction Motors,” IEEE Ind. Appl. Society Annual Meeting,2001.